Magnetic resonance imaging (MRI) is a time-intensive process, as images must be constructed from data collected through numerous individual measurements. However, the integration of machine learning has made it possible to perform imaging with less MRI data, thereby saving both time and cost. A major challenge is the lack of ideal training images for certain applications, such as real-time (moving) MRI, where the images tend to be slightly blurred. An international research team, led by Martin Uecker and Moritz Blumenthal from the Institute of Biomedical Imaging at TU Graz, has developed a method to generate precise real-time MRI images of the beating heart without the need for perfect training images and with minimal MRI data. These advancements could pave the way for more frequent use of real-time MRI in practice.
Calibration of imaging using withheld data
Uecker and Blumenthal trained their machine learning model using self-supervised learning methods. Instead of relying on pre-curated perfect images, the model was trained using a portion of the initial MRI data. The model reconstructed the image from this larger portion and then attempted to predict the second portion of data, which had been withheld. If the prediction was inaccurate, this indicated that the initial image reconstruction was incorrect. The model was then adjusted and improved through several rounds of this process until consistent results were achieved. Through repeated reconstructions, the system learned what constitutes a high-quality MRI image, allowing it to generate accurate images during real-world applications.
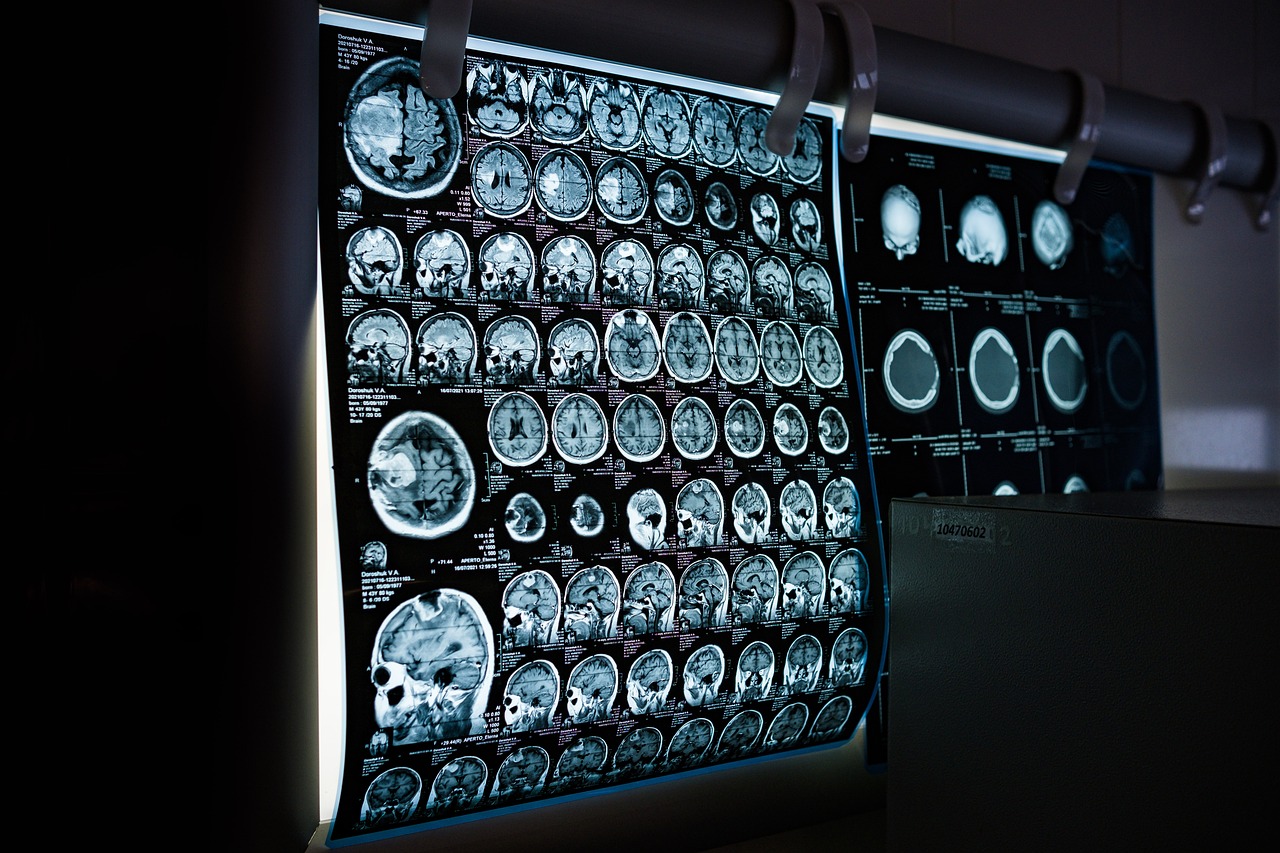
The method is now ready for practical use, though its broader adoption may take some time. This approach has the potential to accelerate many MRI applications, making them more cost-effective. One example is quantitative MRI, where physical tissue parameters are precisely measured and quantified. This technique provides radiologists with accurate data for diagnosis, rather than requiring them to interpret images based on brightness differences. Previously, quantitative MRI measurements have been time-consuming, but this machine learning model has significantly sped up the process without any loss of quality.
Faster and more affordable MRI applications
The research, published in Magnetic Resonance in Medicine, is the result of an international and interdisciplinary collaboration led by the Institute of Biomedical Imaging. The team included Christina Unterberg, a cardiologist from University Medical Centre Göttingen, Markus Haltmeier, a mathematician from the University of Innsbruck, Xiaoqing Wang, an MRI researcher from Harvard Medical School, and Chiara Fantinato, an Erasmus student from Italy. The algorithms and MRI data have been made publicly available, allowing other researchers to replicate and build on this innovative method.
Find the full report here.